5 Success Factors for Integrating Artificial Intelligence into Your Trade Surveillance Program
According to a Chartis research survey The Future of Trader Surveillance, financial services firms are now prioritizing Artificial Intelligence as essential to trade surveillance. In fact, close to 50 percent of firms surveyed listed AI as a key technology driver.
But in spite of AI’s rapid expansion, there are barriers to adoption. According to a separate McKinsey & Company survey, one of the most significant roadblocks is a lack of clear strategy. This is particularly true in the compliance arena where regulatory requirements and internal policies introduce complexities for surveillance and monitoring. When AI is implemented with a lack of understanding and strategic focus, this can lead to less-than-optimal results.
Is your firm considering integrating AI into its surveillance program? Here are five things you need to consider:
1. Start by Defining the Problem
You can’t solve a problem without defining it first. AI is a promising technology for trade surveillance, but it wasn’t intended to solve every problem. For example, supervised machine learning can be very helpful in predicting alert outcomes. Unsupervised machine learning can be equally effective in finding hidden patterns in data. And natural language processing (a form of AI) can be very effective in analyzing trader communications. That said, every surveillance challenge isn’t necessarily best addressed through AI. Consider the following:
How complex is the problem you’re trying to solve?
You don’t need a sophisticated machine learning model when a problem can be solved with simple rules.
How much data is available and how fast can it scale?
Data is essential to machine learning, and not just a little data – a lot of data. That’s one of the reasons that AI-driven product recommendation algorithms used by companies like Netflix and Amazon work so well – an abundance of data. On the flipside, you can’t make reliable predictions off of limited data. If you’re monitoring a small number of traders and that number is not likely to scale up in the future, then AI- powered surveillance solution might not be the best choice for you. However, if your firm employs large number of traders and that number is scaling up fast, AI’s a perfect fit.
How frequently do you need to modify rules and thresholds?
If rules don’t need to be modified often (remain static), then it doesn’t make sense to use machine learning. On the other hand, if the behaviors you’re trying to detect are constantly changing, or you don’t even really know what you’re looking for to begin with, machine learning can provide a detection edge.
What’s your level of tolerance for misclassification?
If Netflix recommends the wrong movie, it’s not the end of the world. But if insider trading goes undetected, that could cause irreparable brand damage.
True – one hundred percent detection accuracy is a myth. But for scenarios where the rules are clear-cut, threshold-based detection is a pretty safe bet. Machine learning may be able to support more types of detection scenarios, but the flexibility could come at the cost of detection accuracy, at least initially (until your data scales).
One benefit of AI-powered surveillance is that it can assign degrees of confidence to alerts, which can significantly cut down on compliance analysts’ workloads by allowing them to focus on alerts that have a higher probability of being true.
2. Have Uniform Processes in Place to Ensure Data Quality
One of the most promising applications for machine learning in compliance is predicting alerts’ outcome based on historical alert data. Financial services firms deal with tons of alerts. A typical tier 1 bank can generate anywhere between 1,000 to 1,500 communication and trade surveillance alerts in a single day. Improving the accuracy of alerts can go a long way toward reducing clutter and resource drain.
Data volume is an important starting point for supervised machine learning, but the biggest challenge really lies in the data quality. Machine learning can only make accurate predictions based on what it learns and knows in the first place. Bad data yields bad results.
In the case of supervised machine learning, humans (namely compliance analysts) play an essential role in ensuring data accuracy. Alerts need to be properly dispositioned. If they’re not, the surveillance system will make future predictions based on bad data.
Ergo, it follows that problems arise when compliance analysts do not follow uniform review processes, or do not close alerts properly, or label them uniformly and accurately. And if supervisors aren’t spot-checking analysts’ work, you may not even know you have a problem in the first place.
If your firm is considering deploying supervised machine learning, you should first make sure there are processes in place to ensure that data will be labeled uniformly and accurately. Prioritizing data quality also means instituting on-going review processes and data quality checks.
3. Make Sure Your AI Models Are Explainable
Many view AI as a ‘black box’ of sorts which data goes into and decisions magically come out of.
Now, put that in the context of the day-to-day decisions you make. You would never let someone else make big personal decisions for you, like what home to buy. You’d want to know that the person had intelligently assessed all of the key factors in making your home-buying decision (e.g. maybe the location, price, condition of the home, property values, taxes, schools in the area).
Similarly, you wouldn’t blindly trust a machine to make consequential compliance decisions for your firm. That’s where AI explainability becomes essential.
When implementing AI, there is a natural tendency to fear AI’s black box nature. But this can be overcome with a solution that incorporates drill down dashboards that provide detailed explanations of every alert prediction.
The solution should provide a view into all the factors that contributed to the alert prediction, (for example, conversation language, conversation topics, the amount/volume of the trade, and so on), along with each factor’s relative weight.
Knowing what went into each alert score can help your compliance analysts make better decisions, and at the end of the day give your firm greater confidence in AI. It can also go a long way toward refining, improving and retraining your models for better accuracy.
4. Keep in Mind What Gets Measured Gets Managed
Whether it’s a human being making decisions, or a machine via AI, if you have no way to measure and track success (in this case, detection accuracy), you could be making the same mistakes over and over again.
The bottom line is – what gets measured gets managed and improved. For this reason, if you’re considering deploying AI as part of your surveillance program, you should look for a solution that offers real time dashboards so you can monitor your supervised machine learning models’ accuracy. This will allow you to see which models are accurate (based on dispositions of alerts) and which are not, how a particular model is performing compared to others, and then use this information to make necessary tweaks and adjustments.
AI explainability can also play an important role in understanding why some models are more accurate than others.
Measuring success isn’t as straightforward with unsupervised machine learning models due to a lack of labeled data. However, predictions made by unsupervised models can and should be reviewed regularly to ensure that the models are in fact making accurate predictions.
5. Don’t Forget Your Current Tools
Like all shiny new things, the impressive capabilities of AI can make other detection solutions appear instantly obsolete. But whether AI is on your short or longer-term surveillance trajectory, there’s a lot of value that can be achieved by dipping one foot in the AI waters, while continuing to tread carefully with the other using existing rules-based surveillance tools.
For one thing, your current surveillance solutions have years of built-in knowledge and complex rules that can’t be instantly emulated by new AI and machine learning-based solutions.
Additionally, as noted above, AI isn’t designed to solve every problem, it requires sizeable datasets, and it takes time for AI to learn your business logic. Switching to an all AI-surveillance environment too fast can introduce risks.
It’s for these reasons I recommend taking a hybrid approach.
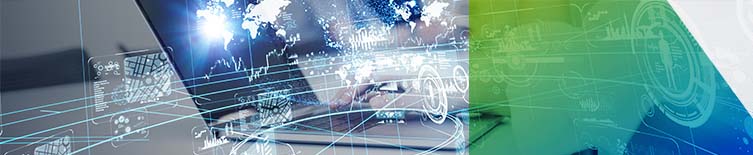
Looking to Enhance Your Surveillance with AI?
As you continue on your AI journey, new challenges will undoubtably come up. As the largest and broadest provider of financial crime, risk and compliance solutions for regional and global financial institutions, NICE Actimize can help with your AI questions and needs.
Our supervised and unsupervised machine learning solutions are already in use at leading financial institutions. Our broad expertise means we’re equipped to help you understand where and how to apply AI and machine learning in a manner that can add value to your surveillance programs. We’re also here to assist with your data quality and other inherent challenges. Finally, our surveillance solutions remove the mystery of AI by providing complete explainability of alerts with drill down dashboards.